Here you can find a consolidated (a.k.a. slowly updated) list of my publications. A frequently updated (and possibly noisy) list of works is available on my Google Scholar profile.
Please find below a short list of highlight publications for my recent activity.
Errica, Federico; Bacciu, Davide; Micheli, Alessio
PyDGN: a Python Library for Flexible and Reproducible Research on Deep Learning for Graphs Journal Article
In: Journal of Open Source Software, vol. 8, no. 90, pp. 5713, 2023.
@article{errica2023pydgn,
title = {PyDGN: a Python Library for Flexible and Reproducible Research on Deep Learning for Graphs},
author = {Federico Errica and Davide Bacciu and Alessio Micheli},
year = {2023},
date = {2023-10-31},
urldate = {2023-01-01},
journal = {Journal of Open Source Software},
volume = {8},
number = {90},
pages = {5713},
keywords = {},
pubstate = {published},
tppubtype = {article}
}

Lomonaco, Vincenzo; Caro, Valerio De; Gallicchio, Claudio; Carta, Antonio; Sardianos, Christos; Varlamis, Iraklis; Tserpes, Konstantinos; Coppola, Massimo; Marpena, Mina; Politi, Sevasti; Schoitsch, Erwin; Bacciu, Davide
AI-Toolkit: a Microservices Architecture for Low-Code Decentralized Machine Intelligence Conference
Proceedings of 2023 IEEE International Conference on Acoustics, Speech and Signal Processing, 2023.
@conference{Lomonaco2023,
title = {AI-Toolkit: a Microservices Architecture for Low-Code Decentralized Machine Intelligence},
author = {Vincenzo Lomonaco and Valerio De Caro and Claudio Gallicchio and Antonio Carta and Christos Sardianos and Iraklis Varlamis and Konstantinos Tserpes and Massimo Coppola and Mina Marpena and Sevasti Politi and Erwin Schoitsch and Davide Bacciu},
year = {2023},
date = {2023-06-04},
urldate = {2023-06-04},
booktitle = {Proceedings of 2023 IEEE International Conference on Acoustics, Speech and Signal Processing},
abstract = {Artificial Intelligence and Machine Learning toolkits such as Scikit-learn, PyTorch and Tensorflow provide today a solid starting point for the rapid prototyping of R&D solutions. However, they can be hardly ported to heterogeneous decentralised hardware and real-world production environments. A common practice involves outsourcing deployment solutions to scalable cloud infrastructures such as Amazon SageMaker or Microsoft Azure. In this paper, we proposed an open-source microservices-based architecture for decentralised machine intelligence which aims at bringing R&D and deployment functionalities closer following a low-code approach. Such an approach would guarantee flexible integration of cutting-edge functionalities while preserving complete control over the deployed solutions at negligible costs and maintenance efforts.},
keywords = {},
pubstate = {published},
tppubtype = {conference}
}
Artificial Intelligence and Machine Learning toolkits such as Scikit-learn, PyTorch and Tensorflow provide today a solid starting point for the rapid prototyping of R&D solutions. However, they can be hardly ported to heterogeneous decentralised hardware and real-world production environments. A common practice involves outsourcing deployment solutions to scalable cloud infrastructures such as Amazon SageMaker or Microsoft Azure. In this paper, we proposed an open-source microservices-based architecture for decentralised machine intelligence which aims at bringing R&D and deployment functionalities closer following a low-code approach. Such an approach would guarantee flexible integration of cutting-edge functionalities while preserving complete control over the deployed solutions at negligible costs and maintenance efforts.
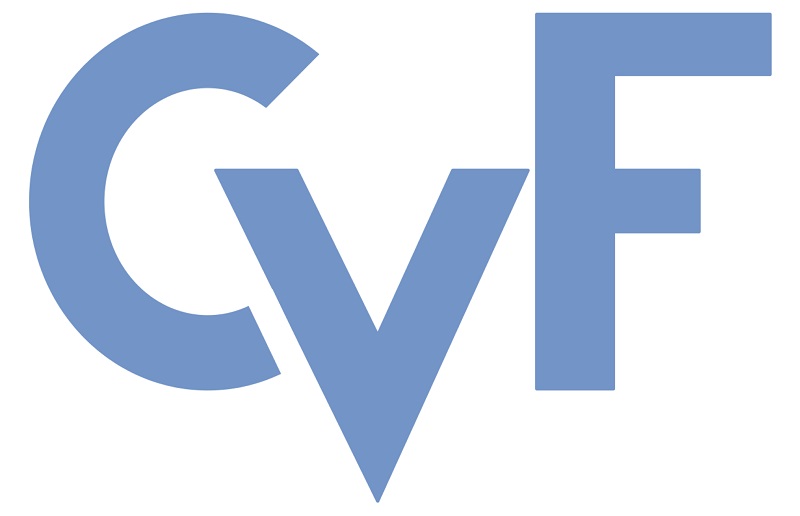
Lomonaco, Vincenzo; Pellegrini, Lorenzo; Cossu, Andrea; Carta, Antonio; Graffieti, Gabriele; Hayes, Tyler L; Lange, Matthias De; Masana, Marc; Pomponi, Jary; van de Ven, Gido; Mundt, Martin; She, Qi; Cooper, Keiland; Forest, Jeremy; Belouadah, Eden; Calderara, Simone; Parisi, German I; Cuzzolin, Fabio; Tolias, Andreas; Scardapane, Simone; Antiga, Luca; Amhad, Subutai; Popescu, Adrian; Kanan, Christopher; van de Weijer, Joost; Tuytelaars, Tinne; Bacciu, Davide; Maltoni, Davide
Avalanche: an End-to-End Library for Continual Learning Workshop
Proceedings of the CVPR 2021 Workshop on Continual Learning , IEEE, 2021.
@workshop{lomonaco2021avalanche,
title = {Avalanche: an End-to-End Library for Continual Learning},
author = {Vincenzo Lomonaco and Lorenzo Pellegrini and Andrea Cossu and Antonio Carta and Gabriele Graffieti and Tyler L Hayes and Matthias De Lange and Marc Masana and Jary Pomponi and Gido van de Ven and Martin Mundt and Qi She and Keiland Cooper and Jeremy Forest and Eden Belouadah and Simone Calderara and German I Parisi and Fabio Cuzzolin and Andreas Tolias and Simone Scardapane and Luca Antiga and Subutai Amhad and Adrian Popescu and Christopher Kanan and Joost van de Weijer and Tinne Tuytelaars and Davide Bacciu and Davide Maltoni},
url = {https://arxiv.org/abs/2104.00405, Arxiv},
year = {2021},
date = {2021-06-19},
urldate = {2021-06-19},
booktitle = {Proceedings of the CVPR 2021 Workshop on Continual Learning },
pages = {3600-3610},
publisher = {IEEE},
keywords = {},
pubstate = {published},
tppubtype = {workshop}
}